Carmona lab
We study patterns of variation across tumors to identify general principles of immune system regulation during tumor progression and response to therapy. We combine data science and computational methods development with high-throughput single-cell and spatial omics technologies with the goal to reveal biological insight and develop predictive models of disease progression and response to treatment. ...
Research projects
Advancing single-cell omics data analysis
Recent advances in single-cell and spatial omics technologies have opened a unique opportunity to explore biological systems at an unprecedented resolution and scale. Our lab develops computational and statistical methods to translate the complex data generated by these technologies into biological understanding. We have created many widely-used open-source computational methods tackling multiple challenges in single-cell data science including semi-supervised integration, cell type classification, reference atlas mapping, gene signature scoring, gene program discovery, tumor microenvironment analysis, and T cell clonal analysis.
Dissecting the tumor microenvironment variation across and within individuals
The tumor microenvironment (TME) is the complex niche surrounding cancer cells, composed of various cell types and molecules that influence tumor growth and treatment responses. In the TME, a striking diversity of immune cells, fibroblasts, endothelial cells, and other tissue-specific cells interact with phenotypically diverse cancer cells and evolve with cancer progression. The TME components and their complex interaction network determine to a large extent disease progression and response to therapies. We use high-resolution spatial and single-cell technologies to study the biological variation that occurs within and between tumors, with the goal to identify general principles of immune system regulation. This approach has the potential to reveal the predominant immune regulation mechanisms underpinning cancer progression and therapy resistance, leading to novel therapeutic targets.
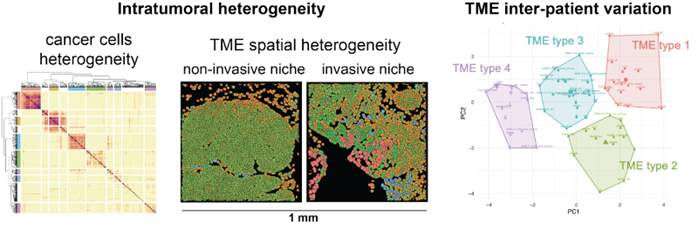
Defining transcriptional and epigenetic reference maps of immune cells
Single-cell transcriptomics and chromatin accessibility profiling allow us to unbiasedly explore cell diversity and to discover cell types, transient cell states and their characteristic gene programs. Robust, expert-curated single-cell maps that can be created with these data modalities have become essential resources as they serve as references to interpret cellular differences across perturbations, conditions, tissues and individuals. A single-cell map is a useful abstraction that allows modeling complex cell heterogeneity as discrete or partially-overlapping cell populations defined by characteristic gene programs activity and chromatin accessibility patterns – and potentially distinct functions. Combining our expertise in bioinformatics and immunology, we generate high-quality reference maps for immune cells that are used by the research community. These are available at our SPICA portal https://spica.unil.ch/.
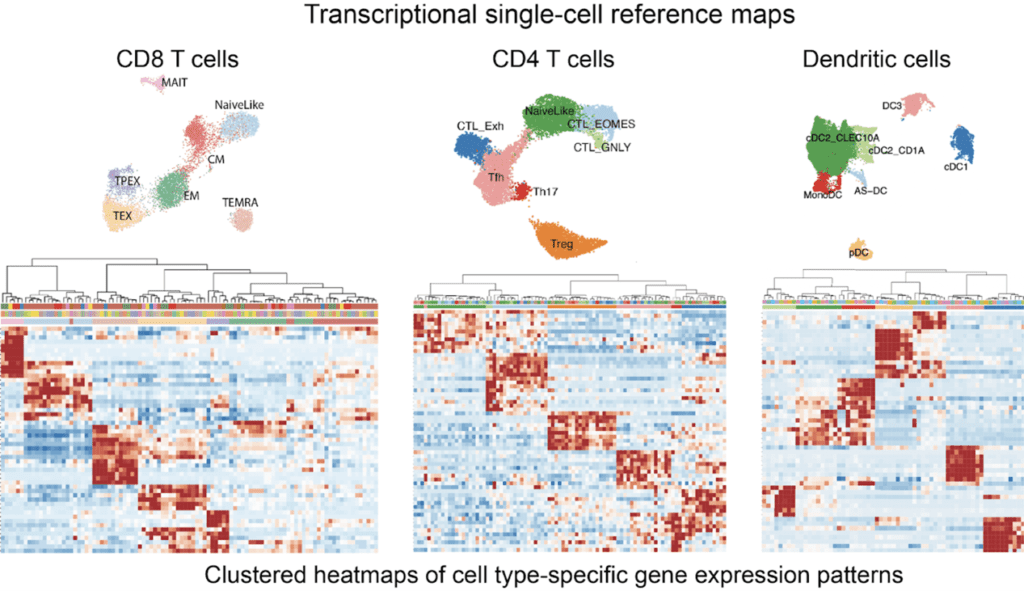
Predictive models for immuno-oncology
Therapies that modulate the immune system, such as immune checkpoint inhibitors, have revolutionized the landscape of cancer treatment. However, only a fraction of patients experience clinical benefit across cancer. Profiling the tumor immune microenvironment offers a great opportunity for the discovery of biomarkers of therapy response and of immune-related adverse events. However, tumor biopsy-based biomarkers face limitations due to tumor heterogeneity and accessibility of various metastatic sites. In contrast, peripheral blood samples present several advantages including enhanced accessibility, feasibility to implementation in clinical practice and possibility of multiple sampling for monitoring of dynamic changes. We study how the peripheral blood is altered by the tumor characteristics at the single-cell level and exploit this knowledge to develop machine learning models to predict disease state and response to therapy.
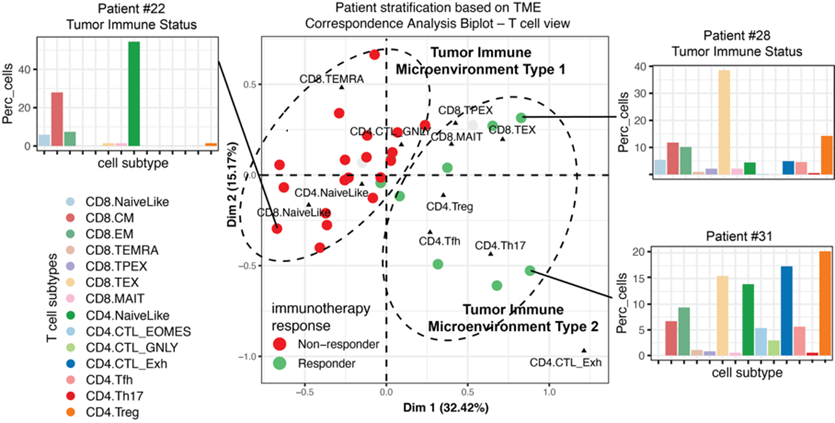
Latest publications
Semi-supervised integration of single-cell transcriptomics data.
Andreatta M, Hérault L, Gueguen P, (...), Gfeller D, Berenstein AJ, Carmona SJ
Nature communications – 2024 Jan 29
IL-10-expressing CAR T cells resist dysfunction and mediate durable clearance of solid tumors and metastases.
Zhao Y, Chen J, Andreatta M, (...), Sun J, Guo Y, Tang L
Nature biotechnology – 2024 Jan 2
T Cell Clonal Analysis Using Single-cell RNA Sequencing and Reference Maps.
Andreatta M, Gueguen P, Borcherding N, Carmona SJ
Bio-protocol – 2023 Aug 20
A CSF-1R-blocking antibody/IL-10 fusion protein increases anti-tumor immunity by effectuating tumor-resident CD8(+) T cells.
Chang YW, Hsiao HW, Chen JP, (...), Ho PC, Chen HK, Yang MH
Cell reports. Medicine – 2023 Aug 15
Team

Santiago Carmona
PhD SNF Ambizione Fellow, Group Leader, Cancer systems immunology, SIB, Department of Oncology UNIL & CHUV
CarmonaLabOther members
Selected Publications
Semi-supervised integration of single-cell transcriptomics data.
Andreatta M, Hérault L, Gueguen P, (...), Gfeller D, Berenstein AJ, Carmona SJ
Nature communications – 2024 Jan 29
T Cell Clonal Analysis Using Single-cell RNA Sequencing and Reference Maps.
Andreatta M, Gueguen P, Borcherding N, Carmona SJ
Bio-protocol – 2023 Aug 20
Orthogonal cytokine engineering enables novel synthetic effector states escaping canonical exhaustion in tumor-rejecting CD8(+) T cells.
Corria-Osorio J, Carmona SJ, Stefanidis E, (...), Luther SA, Irving M, Coukos G
Nature immunology – 2023 Apr 20
A CD4(+) T cell reference map delineates subtype-specific adaptation during acute and chronic viral infections.
Andreatta M, Tjitropranoto A, Sherman Z, (...), Kelly MC, Ciucci T, Carmona SJ
eLife – 2022 Jul 13
scGate: marker-based purification of cell types from heterogeneous single-cell RNA-seq datasets.
Andreatta M, Berenstein AJ, Carmona SJ
Bioinformatics (Oxford, England) – 2022 Apr 28
SPICA: Swiss portal for immune cell analysis.
Andreatta M, David FPA, Iseli C, Guex N, Carmona SJ
Nucleic acids research – 2022 Jan 7
UCell: Robust and scalable single-cell gene signature scoring.
Andreatta M, Carmona SJ
Computational and structural biotechnology journal – 2021 Jun 30
Interpretation of T cell states from single-cell transcriptomics data using reference atlases.
Andreatta M, Corria-Osorio J, Müller S, (...), Cubas R, Coukos G, Carmona SJ
Nature communications – 2021 May 20
STACAS: Sub-Type Anchor Correction for Alignment in Seurat to integrate single-cell RNA-seq data.
Andreatta M, Carmona SJ
Bioinformatics (Oxford, England) – 2021 May 5
Deciphering the transcriptomic landscape of tumor-infiltrating CD8 lymphocytes in B16 melanoma tumors with single-cell RNA-Seq.
Carmona SJ, Siddiqui I, Bilous M, Held W, Gfeller D
Oncoimmunology – 2020 Mar 12
Related news
Events
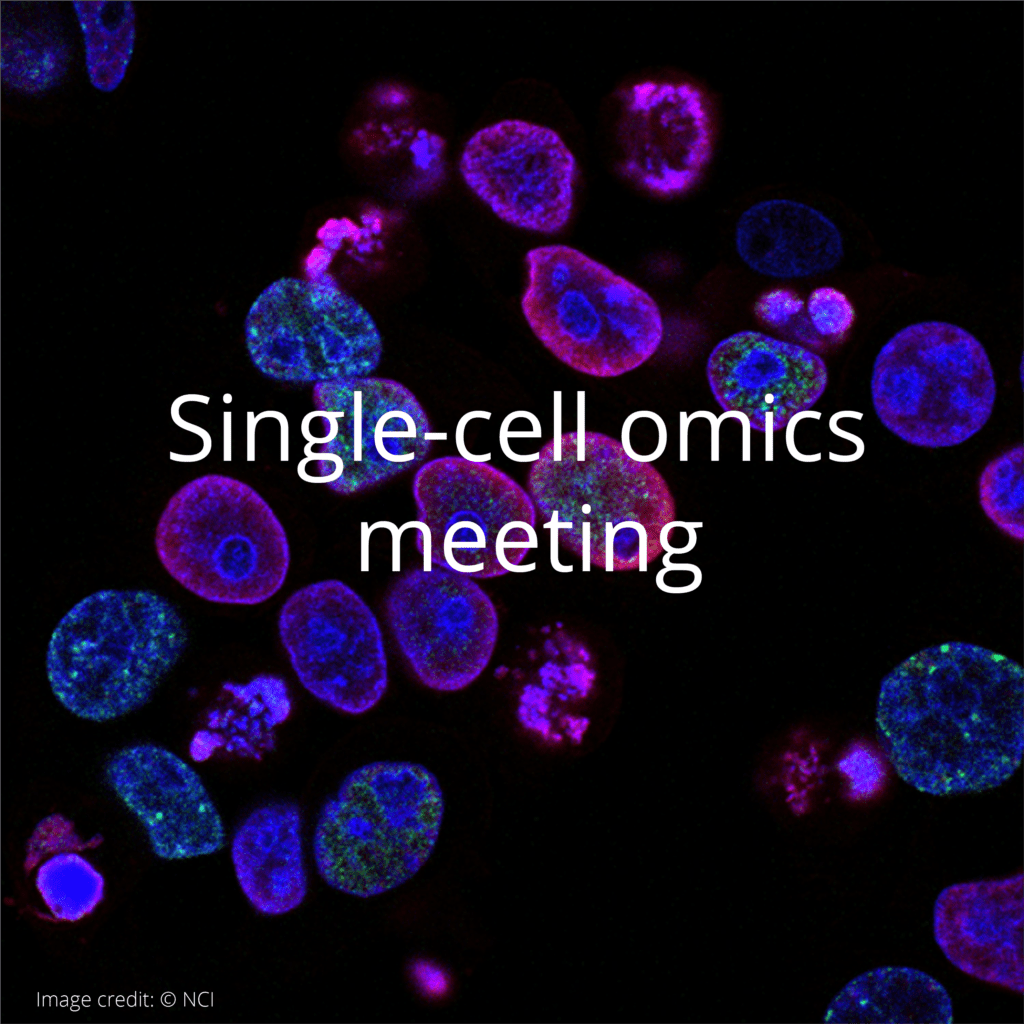
AGORA Single-cell omics meetings
Events
AGORA Sustainability Day
Events
SYIS Seminar Series | June 20th
Events

AGORA PRS | April 23rd
Events

AGORA PRS | December 12th
Events
Visiting scientist seminar, Dr. Kieran McGourty | December 8th
Events
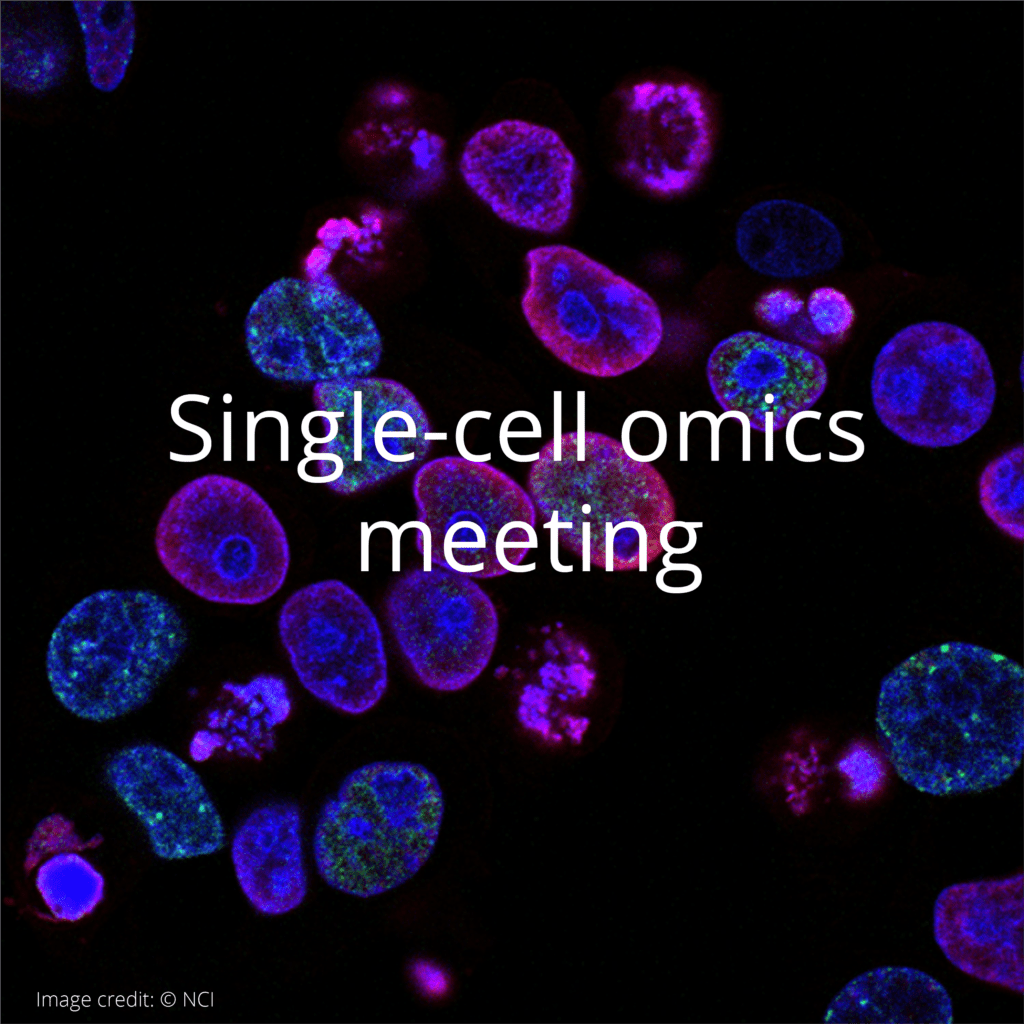
AGORA Single-cell omics meetings
Events
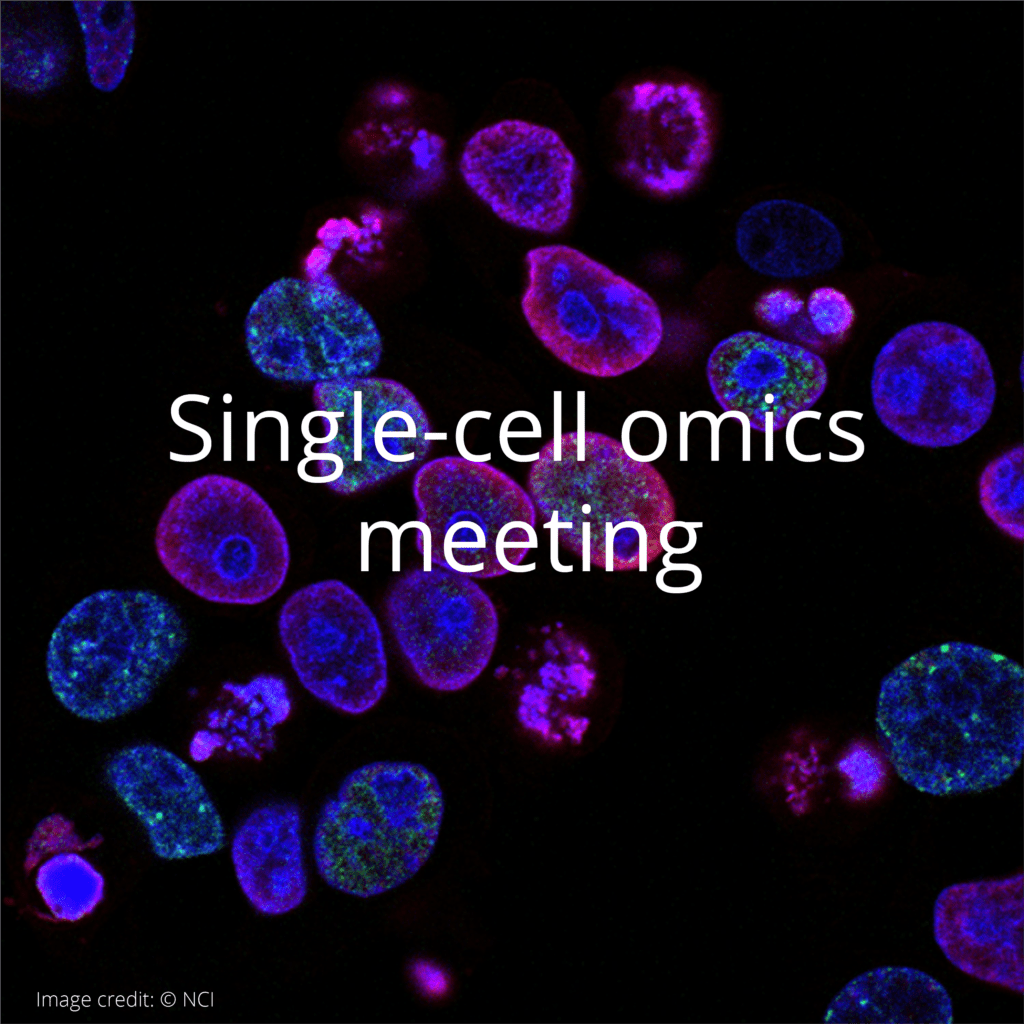